SubtlePET
AI solution for PET scan optimization
SubtlePET™ is a deep learning solution enabling nuclear medicine practices to reduce acquisition time on existing machines and/or reduce the dose injected during acquisition. For nuclear doctors, improving diagnosis and management necessarily involves constantly optimising image quality.
Starting with a poor-quality image, SubtlePET corrects and sends back an image of equivalent quality to one taken under standard acquisition conditions, directly to the radiologist’s reading tools.
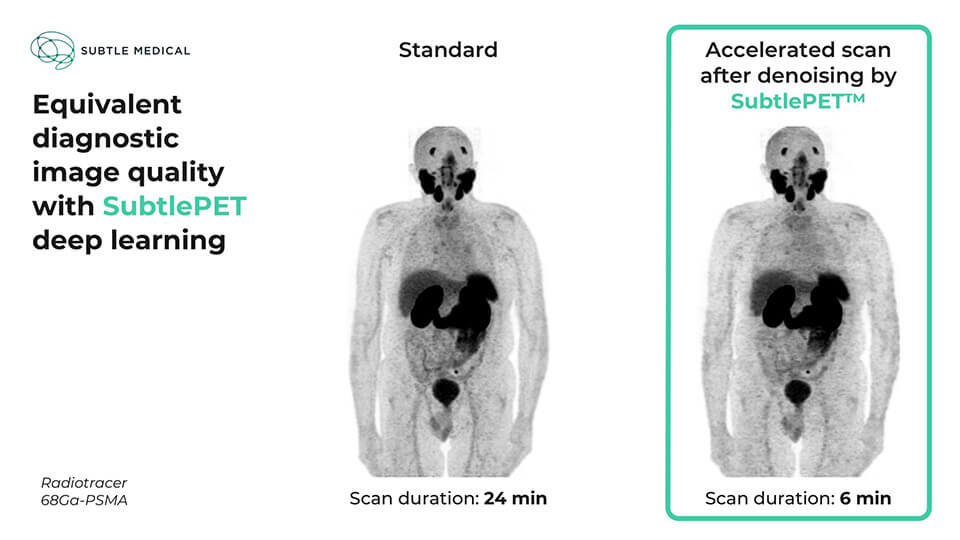
Reduced acquisition time
SubtlePET improves quality of images taken up to 4X faster. The image returned by SubtlePET is of standard clinical quality.
Reduction of injected dose of FDG, FCH, FDOPA, Ga-PSMA…
SubtlePET improves quality of images taken with a reduced injected dose. The image returned by SubtlePET is of standard clinical quality.
Compatible with all PET scan models and supplier
SubtlePET delivers the exam directly into the radiologist’s reading tool and therefore fits seamlessly into the existing infrastructure.
- PET scan
- nuclear medecine
- scanner
- low dose
- full-length scan acquisition
- denoise
- scarce nuclear tracers
Save time, reassure your diagnosis and streamline your workflow with Incepto
Testimonial
The quality of the images improved by the Subtle tool makes them indistinguishable from images obtained by conventional means. In addition, the results are free of artifacts and lesions can be easily identified. I can already see how the tool will improve both our workflow and the patient experience.
Peter Giuliano,
Director of the Department of Nuclear Medicine
HOAG Hospital (California – USA)
Publications
- Quantitative Standardized Uptake Value Evaluation of 4x Faster PET Scans Enhanced using Deep Learning – A Chaudhari, PhD; P Gulaka, PhD; T Zhang; S Srinivas, MD, PhD; G Zaharchuk, MD, PhD; E Gong, PhD, Stanford.
- 200x Low-dose PET Reconstruction using Deep Learning – Junshen Xu*, Enhao Gong*, John Pauly, Greg Zaharchuk.
- Accelerating Whole-Body PET Acquisitions Using Deep Learning: External validation on foreign country data – Jose Leite, Gustavo Tukamoto1, Akshay Chaudhari, Praveen Gulaka, Enhao Gong, GregZaharchuk, Igor Rafael Martins dos Santos, Flávia Paiva Proença Lobo Lopes, Felipe Campos, Kitamura.
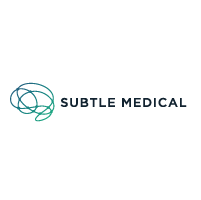
Subtle Medical was founded by a Californian team from Stanford led by Enhao Gong (PhD in Engineering) and Greg Zaharchuk (MD, PhD). With their dual expertise in clinical and data science, their team aims to revolutionise medical imaging using artificial intelligence. Their efforts are focused on image acquisition and processing, with three products developed to date in PET-scanner and MRI modalities.
The SubtlePET medical device is a regulated healthcare product bearing the CE mark, Class I. Manufacturer: Subtle Medical.
SubtlePET is a diagnostic aid and cannot be used on its own to recommend medical management for diagnostic purposes. Please read the user manual carefully. Please contact contact@incepto-medical.com for further information. Last update : 03/11/2022